Digital Twins: A new Technology Trend
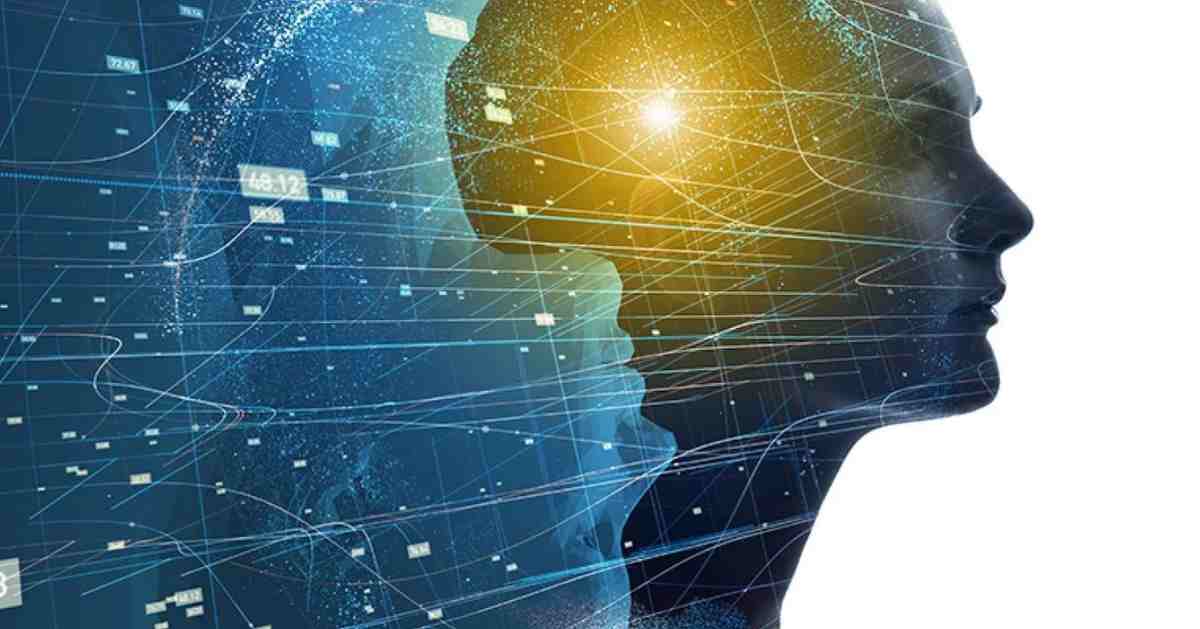
The Digital Twins are presented as a disruptive technology in the simulation and analysis of industrial processes, capable of maximizing the benefits of digital plant transformation, a transformation in which many companies are already involved and which will intensify in the coming years. The Digital Twins are digital representations “live” devices and processes that make up a factory, connected to the real system that represented by “cyber – physical systems” (CPS ). With live plant information, the history of operations and maintenance, and the application of Machine Learning techniques, it is possible easy to Buy Assignment Online obtain a high precision model whose behaviour closely resembles that of the real system.
Consequently, we achieve a protected and safe environment for experimentation, being able to detect problems before they occur, plan maintenance tasks avoiding unexpected stops, build new more efficient operating scenarios (OEE), develop new business opportunities and new manufacturing plans, or even make future forecasts. However, the Digital Twin is still a development concept that also presents several technological barriers for its adoption in the industrial fabric. On the one hand, there is a technical difficulty to massively monitor and digitize processes in the industry, with a wide variety of equipment, isolated legacy systems, field buses, proprietary protocols, as well as a strict integration architecture and industrial automation.
On the other hand, the systems currently used in the industry are unable to store and process the volumes of data necessary to create and evolve the Digital Twins, which really represent the behaviour of the physical elements, and not only their characteristics and their state. This is linked to the technological complexity required to go beyond digital representations and move towards multiple simultaneous digital copy management scenarios, with greater capacity to evaluate alternative scenarios.
The DIGITAL TWINS project began in 2017 focusing on these technological barriers, through the use of the latest advances in technologies of the Internet of Things Industrial (IoT) and in learning techniques based on Big Data. The consolidation of the Internet of Things (IoT) in Industry 4.0, in addition to the new advances in high-volume warehouse techniques and automatic learning through Big Data Analytics, has enabled the development of new concepts within the industry, such as case of the Digital Twins.
The concept of Digital Twins still presents technological barriers for its full development, and therefore does not allow its adoption in the industrial fabric in general. On the one hand, there is a technical difficulty to massively monitor and digitize processes in the industry, with a wide variety of equipment, isolated legacy systems, field buses, proprietary protocols, as well as a strict integration architecture and industrial automation. On the other hand, the systems currently used in the industry are unable to store and process the volumes of data necessary to create and evolve the Digital Twins, which really represent the behaviour of the physical elements, and not only their characteristics and their state.
This is linked to the technological complexity required to go beyond digital representations, and advance towards multiple simultaneous digital copy management scenarios, with greater capacity to evaluate alternative scenarios.
Digitization of plant deploy& forget
Through the application of IoT and Cyber-Physical Systems (CPS) , ITI is working on a proof of concept of a plug & play system to digitize elements in plant (old and modern machinery, tools, people, products etc.) in an agile and minimally intrusive way. This system, baptized as “CPS deploy & forget”, is specially designed for a quick and easy start-up, without the need for communications and energy infrastructures, and for a transparent integration with the plant systems through the new architectures and protocols of Industry 4.0. The system uses fog-computing techniques to absorb all the complexity involved in the diagnostic and maintenance tasks of the equipment and its communication networks, as well as a simplified system for configuration and start-up. In this way, deploy this system in different locations to perform specific audits.
Its power lies in the “digitizers”, intelligent and autonomous elements that do not require their own communications infrastructure, since the nodes themselves have the ability to self-organize among themselves and solve many of the maintenance problems that until now had to solve the plant staff. These digitizers have a set of integrated sensors to measure different parameters such as temperature, humidity, vibration or energy consumption, but also allow the connection of sensors, actuators and additional interfaces, which extends its use to virtually any operation required in industry.
Big Data Analytics for the construction of Digital Twins
A requirement for the construction of a Digital Twin is to use all the data that is available. However, the large amount of data generated by a plant in continuous poses a challenge in terms of both storage and processing. This fact requires the application of Big Data Analytics technologies, to efficiently manage data in real time, combined with historical and context information (logistics, sales, warehouse, environment etc.). On the other hand, for the creation of the predictive model that supports a Digital Twin, the application of cutting-edge techniques in the field of Machine Learning is necessary. Only by introducing both facets, it is possible to create a Digital Twin that provides precise simulations from both its current operations and past and future states.
The results of the project will be transferred and promoted to the companies of the sectors involved (manufacturing industry, ICT, industrial equipment and sensors, engineering, etc.), and will allow them to create new products, generate employment, improve the professional qualification of their employees, increase their profits and competitiveness, improve their innovation capacity and increase their industrial diversification.