Common Use Cases for Parallel Coordinate Plots
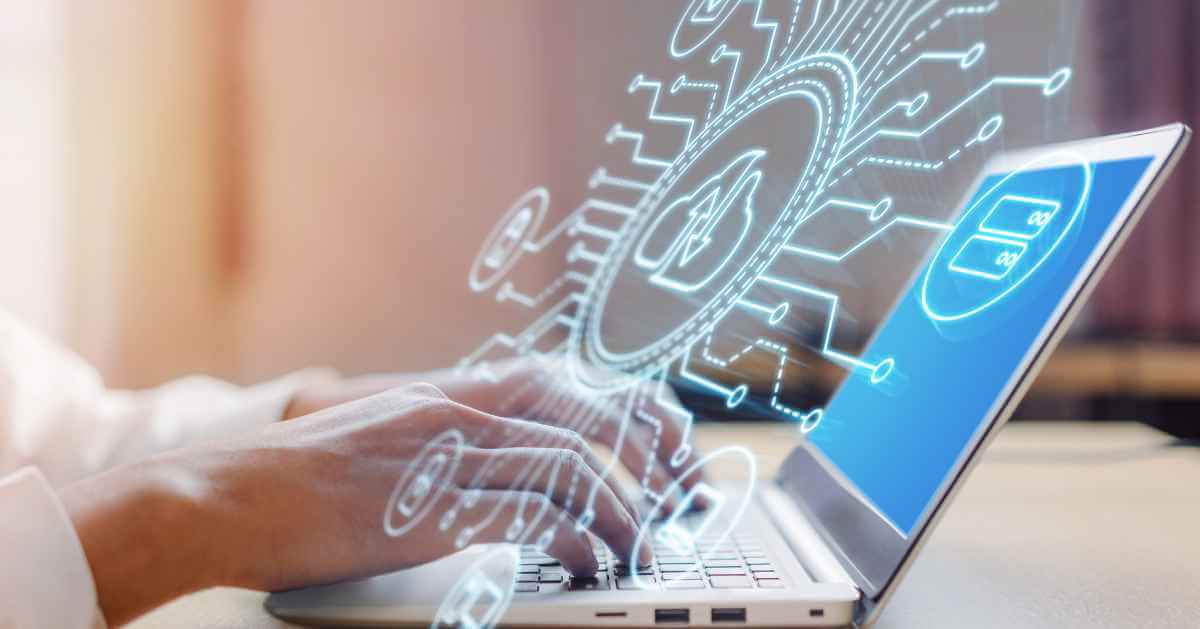
Parallel coordinate plots have become increasingly popular among data analysts and scientists. However, the extent of their application may still be vastly unappreciated. Their complex appearance may seem intimidating, yet understanding and utilizing these charts can drastically improve the comprehensibility of high-dimensional data. Keep reading for a detailed discussion of these intriguing tools.
Understanding the Basics of Parallel Coordinate Plots
The parallel coordinate plot is essentially a multiscale diagram data analysts use to visualize multidimensional numerical data. The method involves representing each data instance with a polyline that intersects a sequence of parallel axes corresponding to the dimensions of the data.
This complex charting system is famous for its ability to cater to a large number of variables while retaining simplicity in visualization. It facilitates the extraction of comprehensive results from high-dimensional datasets. Essentially, these plots provide a profound understanding of data patterns and correlations.
The representation of multivariate data is a crucial aspect of a wide array of disciplines, including machine learning, computer vision, and pattern recognition. Analysts turn to a visualization technique known as a parallel coordinate plot to overcome the difficulty of understanding data in high dimensions.
Emphasizing the Benefits of Using Parallel Coordinate Plot
Parallel coordinate plots have proven to be an invaluable technique when dealing with multivariate data. It distinctly demonstrates the interrelationship between variables and even allows trend identification.
One prominent benefit is its ability to explicate clusters of data points. This elucidates patterns and relationships that simple graphing tools might miss. Such data classification empowers scientists and analysts to make informed decisions and predictions.
These plots reduce the cognitive workload in interpreting complex, high-dimensional data. This enables data-driven storytelling, making the information more accessible and understandable for diverse audiences.
Investigating Relationships With Parallel Coordinate Plots in Data Analysis
Identifying trends and patterns is a key strength of parallel coordinate plots in data analysis. These plots can articulate complex data structures for large datasets of numerous dimensions.
Analysts can identify whether the data points move in a similar direction through the linear representation of variables, indicating a strong relationship. Outliers and anomalies can also be detected, providing a comprehensive picture of the data landscape.
A crucial characteristic of these plots is the expression of correlations. For example, if two variables share a direct relationship, their trajectory lines will maintain a similar pattern across both axes. Conversely, an inverse relationship will reflect lines crossing between the two axes.
The simple yet effective visualization of these aspects simplifies data interpretation and aids in data cleaning processes.
Cases Beyond Data Analysis: Further Applications of Parallel Coordinate Plots
Beyond data analysis, parallel coordinate plots have found use in other fields, including geophysics, computational fluid dynamics, and pharmaceutical development. For example, these plots have been used in geophysics to interpret multivariate borehole data.
Pharmaceutical development has seen the adoption of this technique to comprehend and monitor process parameters, reducing variability and ensuring the consistent production of high-quality drugs.
In computational fluid dynamics, parallel coordinate plots have been leveraged to visualize multidimensional results from simulations. Such applications highlight the adaptability and utility of these plots across an expanding range of disciplines.
The potential for further application of this technique in diverse fields is substantial, bearing testament to the versatility of this powerful visualization tool.
The parallel coordinate plot presents an efficient, insightful means to visualize complex data. Detailed exploration of its potential can greatly enhance how data is interpreted and understood. With ongoing developments in this area, we can anticipate even more advanced tools and techniques emerging in the near future.