Tips for the Best Data Annotation service in the market
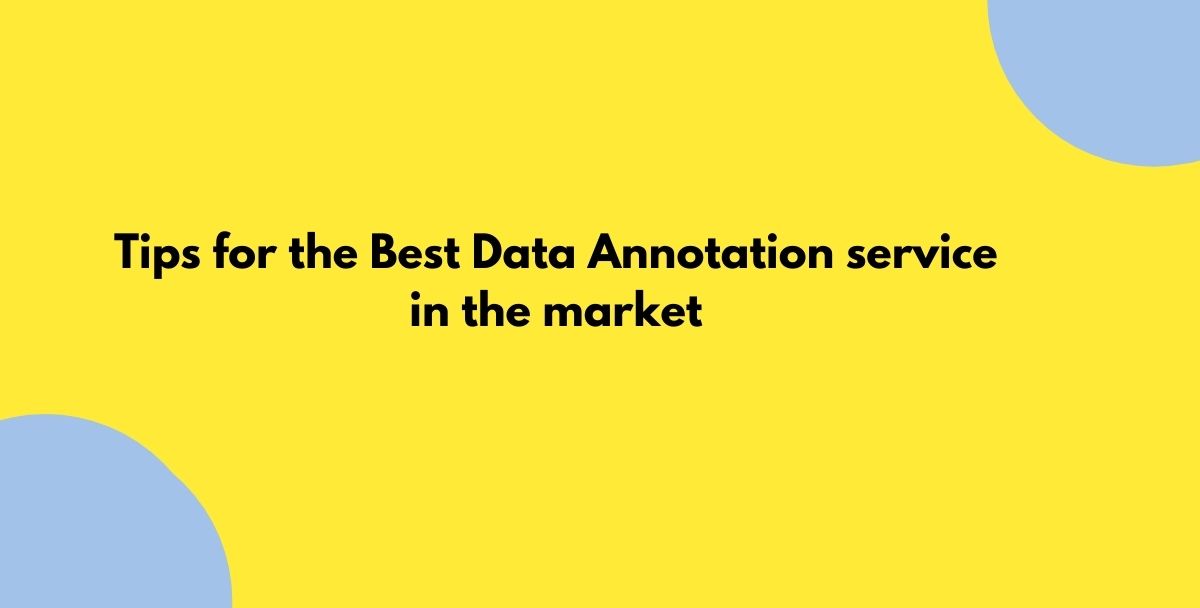
Data Annotation service is used on diverse objects to make them identify and understandable to machine learning algorithms. The data is stored in a large database and accessed by various machine learning algorithms in order to train the systems. However, it’s very hard for people to understand the huge amount of data. Hence, it’s necessary for the further progress of the machine learning (ML) industries like face recognition, automated driving, aerial drones, etc.
Data quality assurance is one of the primary reasons for data Annotation services. It helps in the decision making process. When the project is going through the quality control phase, data quality checks play a vital role. This way, the project manager can easily track down bugs, defects, or broken links. Moreover, a regular check process helps in checking the new features that are added to the system for machine learning projects. Such features can help in improving the quality of the product or service and can bring remarkable results.
One important tool used in the data Annotation service is the code analysis tool. This tool provides high-level insights and helps in making the overall code easy to understand. A good code analysis tool should be written in high-level languages like Java or MATLAB or C++. Such software allows one to quickly analyze the data and suggests the right way to label the data in the system. Another important thing to note in the context of Java code analysis tools is that it should support multi-user capability and also be written in such a way that it becomes easy to extend the tool in the future.
Data labelling with mpls is another tool used in the data Annotation service. This process is called as MLQ or Large Layer Smooth Prediction. In this process, the developer uses the smoothest layer of the data sets that gives a good representation of the data sets. The accuracy of such techniques depends upon the skill and expertise of the developer. The developer uses a network of expert consultants for performing MLQ process.
The other tip for the best data labelling for the 21st century is to avoid the use of complex software like batch processing applications. Such applications are good when it comes to large data sets but not when it comes to small data sets. The best tips for data labelling for the future are provided by the data vendors who have been successful in the field. These vendors provide the best tips and tricks for a smooth data labelling experience in the present time. Such vendors provide training seminars and offer the best services for building an intelligent labeling system for the enterprise.
Data labelling is a very important process in machine learning training data sets. With the help of the tools, the classification of the data can be done easily. The machine learning training data sets are very important especially when it comes to deployment and integration. This means that with the help of machine learning training data labelling can be done easily in a few steps. With the help of technology, the machine learning processes can be automated and the classification of the data can be done properly.
Data Annotation Service and Machine Learning Algorithms
A Data Annotation Service is a web-based service where structured information is tagged with metadata and then categorized to form a new record or set of records. This allows users to easily identify the field or term they wish to have data on and then get the information directly from a database or application. What are the benefits of using these services?
The primary benefit of a data Annotation service is to make data analysis easier. Great service will allow one to easily classify an image or text based on word frequency, grammatical class, spellings, and even cultural influences. For example, if one has access to an ML system and wishes to label images with a tag such as “red face” then they can do this easily and instantly from the ML platform itself.
Not only does the data classification benefit the end-user but also the developer who has designed the web application. It takes less time to write code and more time to run the application when everything is already pre-structured and labelled.
Another benefit is that it reduces development time. With the use of a data analysis tool, it is possible to create training data sets in a matter of minutes and create an easy to analyze the association between variables by just using the text tag or text string alone. The text tag can be typed and written in any text editor such as Microsoft Word or Google Docs (Git) and then saved into a data dictionary.
Once saved it can be referenced in the analysis tool to create correlated variable analysis. This type of analysis is not possible without the help of text analysis tools. It will reduce the amount of time necessary to train a predictive model by many orders of magnitude and will allow one to reach higher and deeper levels of ML performance.
By using a data analysis tool, one can reduce the time needed for training a model to work by leveraging on already trained data. This also enables one to leverage on already high-quality data labelled with complex Vectors or labels such as transcripts or proteins. This means that they become able to provide better and more relevant answers to their users. This then allows for innovative data management and better quality results in terms of product quality, safety, or value.
Finally, with the help of a data labeller` or data analysis tool, it becomes easier for new insights to emerge from the huge amounts of unlabeled data. With the right combination of a data quality service, creative coding in ML domain applications, and ai training, you will be able to create machine learning models which are able to churn out reliable and accurate results in a matter of minutes. It is important to note however that even these models will not be able to generate enough revenue for a business to make it.